How could generative AI improve built environment projects?
Generative AI seems to hold immense promise, allowing straightforward, natural language descriptions to generate complex outputs. In this article, we explore specific scenarios in which generative AI could improve productivity and work quality within real estate and infrastructure.
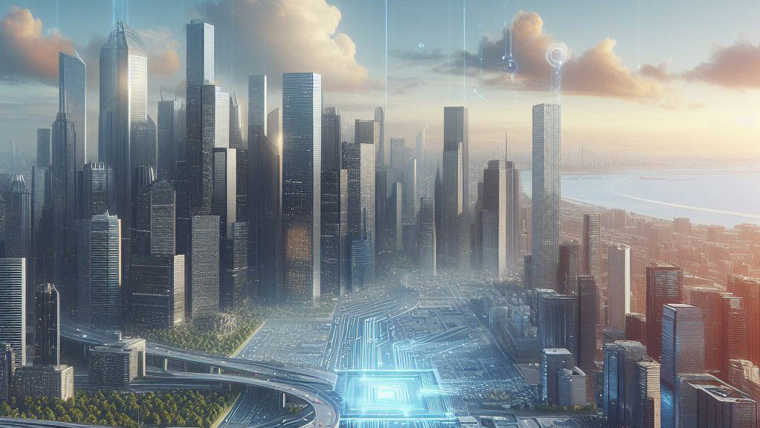
Since the emergence of ChatGPT in November 2022, artificial intelligence and, more specifically the potential of generative AI, has dominated headlines. The ability to input a prompt and obtain a creative textual, graphical, or acoustic output has profound implications for almost every industry and discipline. And in time, that likely includes real estate and infrastructure.
At first glance, generative AI appears to possess incredible potential: enabling simple, natural language descriptions to produce sophisticated outputs. Yet the built environment industry operates within parameters that have historically prevented it from innovating and technologically evolving at the same pace as other design and engineering-based sectors like automotive, aerospace or electronics. This high friction to innovation makes it complicated to guess the scope of adoption, change, and impact this technology might have in our industry.
But again, the promise of generative AI seems tremendous, even in the slow-moving built environment. In this piece, we dive into particular use cases where generative AI may enhance productivity and quality of work in the real estate and infrastructure sector.
Finding a role for gen AI in the built environment
Currently operational generative AI tools like ChatGPT and Midjourney can perform tasks incidentally useful to built environment practitioners. For instance, optimising report writing or generating nonessential yet nice-to-have visuals. However, the impact of these is mostly low and normally inadequate for the rigorous processes of architecture and engineering, and professional design more broadly.
As difficult as it is to imagine currently available tools will transform work in the built environment, we can imagine that subsequent generations may soon be able to address daily tasks and ultimately unlock productivity.
Rational ideation and solution generation
Text-to-image generators are already used to generate geometries, patterns, and textures. However, the applicability of these tools is currently limited to low-stakes artistic ideation. As an industry, we already have incredibly powerful parametric design tools, whose algorithms are carefully scripted to generate workable options based on mathematical and scientific rules and within defined ranges.
The headline grabbing large language models (LLMs) by contrast produce outcomes based on probabilistic estimations which can fail to follow simple mathematical or mechanical rules. This leads to the ‘hallucination’ problem where outputs are confidently inaccurate about facts, dates or arithmetic; results can be visually or linguistically impressive, without actually being in any sense ‘useful’.
Built environment solutions are constrained and defined by parameters like required function, budget, site location, aesthetical aspirations, ground conditions and material or plant availability. These are considerations that current generative AI tools struggle to understand or respond to. This could be the result of limitations like insufficient project data availability or quality, or incapability to process graphical technical information.
To overcome these limitations, we would need to see data enrichment and further model training which could lead to tools that generate logical, workable outputs relevant to real estate and infrastructure designs. Or we might end up using a hybrid approach where gen AI models are supplemented with rule-based parametric.
...the built environment industry operates within parameters that have historically prevented it from innovating and technologically evolving at the same pace as other design and engineering-based sectors like automotive, aerospace or electronics.
Eiki Homma
Senior Engineer
Technical drawing generation and review
Even after decades of ever more sophisticated CAD software, architects, engineers, technicians, and consultants still spend an enormous amount of time working with drawings. That is, producing, curating, annotating, checking, reviewing, and revising technical drawings that will allow for the costing, fabrication and construction of the real estate or infrastructure asset. Perhaps there is a role for generative AI in this realm.
At present, there are only a few image-to-image generators which appear to be capable of processing graphical inputs. However, none of these appear to yet be able to process the technical level of information in built environment design packs ith annotations like dimensions, levels, member sizes, materials, etc. In the same way generative machine learning models cannot yet process this type of data, they cannot yet produce it.
However, this is a gap that could, with investment, training and technical development, be closed. Once generative AI becomes capable of streamlining drawing production similarly to how it already does with reports, sectorial productivity should dramatically increase.
Automatic regulatory and best-practice checks
Documentation and reporting is another area of considerable time and effort in the industry. Here, the fit for generative AI seems stronger and closer at hand. Generative text generating tools, especially LLMs, can search the web or internal server and write a synthesised answer. Moreover, they can summarise, rephrase, analyse, and correct text the user may have directly input in the prompt.
In essence, LLMs can be used as relatively credible reasoning search engines and text generators. Both functions are already relevant for reporting; however, the two could be combined to further streamline the checking stage of the design process or to spot errors or anomalies more frequently throughout development.
A generative AI feedback tool for designers trained on the various international, national or local laws, regulations and codes that building and infrastructure need to comply with would be invaluable. Furthermore, industry design guides and manuals which designers use to support and benchmark their work could be included in the mix.
Nonetheless, codes and manuals are text-based but also feature tables, charts, equations, or diagrams that current generative AI models cannot adequately process. Again, we can hope and anticipate that both elements will become interrogable datasets for training future tools.
Once generative AI becomes capable of streamlining drawing production similarly to how it already does with reports, sectorial productivity should dramatically increase.
Eiki Homma
Senior Engineer
Generative AI’s promise for the built environment
As we have seen in its initial public evolution, most generative AI tools are still predictably aimed at consumer, media and entertainment, self-driving, human resources and other less technical sectors. However, architecture, engineering and construction professionals will likely become beneficiaries in time. Designers should not settle for the low innovation status quo. Generative AI will bring opportunity for the innovators who will adopt and advance the technology, built environment firms and the public who will ultimately enjoy the product: better real estate and infrastructure.
Get in touch with our team